Correlation processing of image grayscale in veterinary B-ultrasound system
Grayscale co-occurrence matrix, the grayscale co-occurrence matrix of veterinary B-ultrasound images reflects the comprehensive information of image grayscale about direction, adjacent intervals, and change amplitude. It is the basis for analyzing the local pattern structure and arrangement rules of veterinary B-ultrasound images. As the feature quantity of texture analysis, it is often not directly applied to the calculated grayscale co-occurrence matrix, but the texture feature quantity is extracted on the basis of the grayscale co-occurrence matrix, which is called the secondary statistic. The grayscale level of a veterinary B-ultrasound image is generally 256 levels. Such too many levels will lead to a large grayscale co-occurrence matrix and a large amount of calculation. Therefore, before calculating the grayscale co-occurrence matrix, it is often compressed to a lower level.
In the veterinary B-ultrasound texture image, the statistical law of the grayscale appearance of a pair of pixels separated by a certain distance in a certain direction should be able to specifically reflect the texture characteristics of this veterinary B-ultrasound image. This statistical law can be described by the grayscale co-occurrence matrix of a pair of pixels, and then some parameters can be calculated from it to quantitatively describe the characteristics of this texture.
Veterinary B-ultrasound EVO
According to the texture characteristics of normal animal liver, fatty liver, and cirrhosis in veterinary B-ultrasound images, the grayscale co-occurrence matrix feature description method is applied to extract feature parameters of the regions of interest of different veterinary B-ultrasound images, and the laws reflected are compared and verified with the classification method. Through the reasonable allocation of various parameters of the BP network, the automatic recognition and classification of animal tissue textures in veterinary B-ultrasound can achieve good resolution. Reasonable selection of the sampling interval and secondary quantization grayscale of animal liver texture has been made and good results have been obtained. In terms of texture feature extraction, some people are studying the use of wavelets with good local descriptions in frequency and space domains for texture analysis.
link: https://www.bxlimage.com/nw/324.html
tags: veterinary B-ultrasound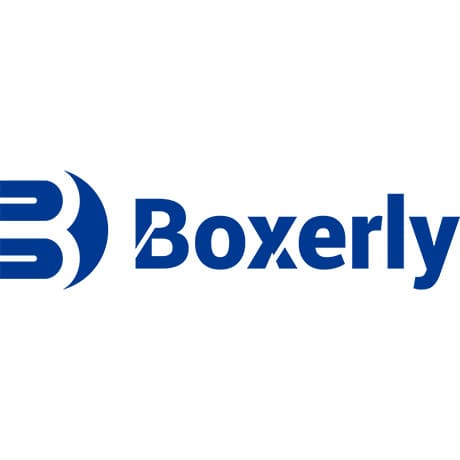
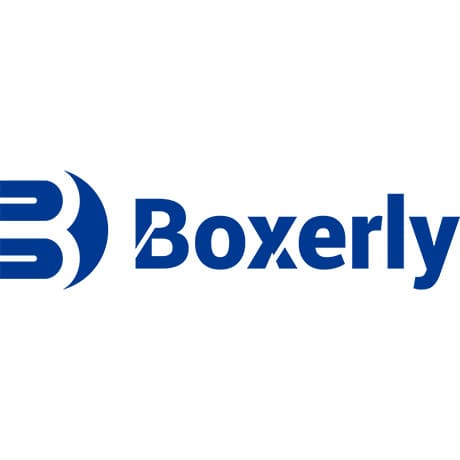
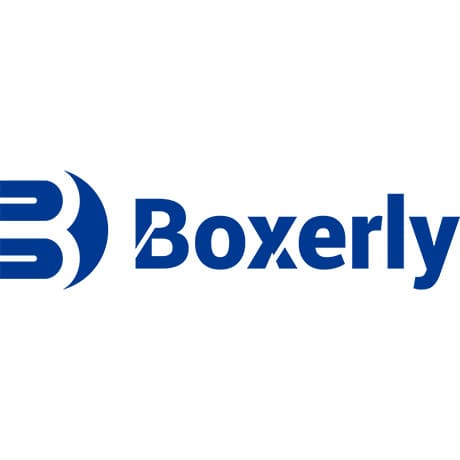
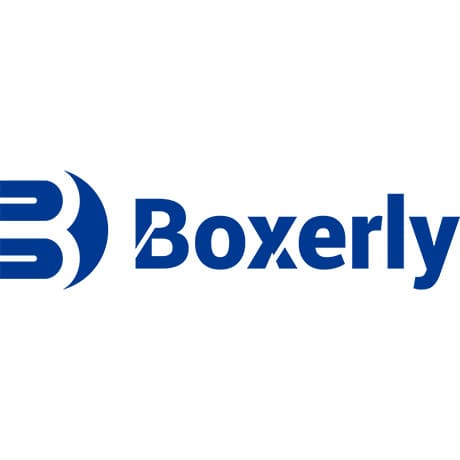