Application of Backfat Analyzer in Image Segmentation of Cattle Carcass Eye Musclec
Image segmentation is a key technology in image processing and analysis. The real-time performance of backfat analyzer in beef automatic grading system requires more in-depth research on beef image segmentation methods, continuous improvement of existing methods, and proposal of new methods to develop towards faster and more accurate direction.
In recent years, with the emergence of new theories and methods for image segmentation in various disciplines, many image segmentation techniques have emerged that combine new theories, tools, and technologies. Some of these technologies, such as the backfat analyzer, have great potential for segmentation of bovine carcass eye muscle images and should be given special attention.
The backfat analyzer proposes a binarization method based on the expected value of statistical features of image grayscale distribution as the threshold. This is a segmentation method that uses the expected grayscale value as the global threshold. Compared with the grayscale difference histogram method, differential histogram method, non equivalent entropy method, and minimum ambiguity method, this algorithm has significantly better performance than the grayscale difference histogram method and differential histogram method, and is comparable to the minimum ambiguity method. However, both the algorithm and execution time are superior to the minimum ambiguity method.
Backfat analyzer for detecting beef cattle
The backfat analyzer applies fractal dimension to grayscale image binarization and studies the selection method of Gibbs model parameters when using a layered Gibbs random field model for image binarization. By defining the relationship between Gibbs model parameters and fractal dimension, adaptive selection of Gibbs model parameters has been achieved. This method can effectively preserve the details of the original image in binary images. In addition, by setting the weight factors of Gibbs model parameters in different orientations of the image, such as horizontal, vertical, and diagonal, directional details can be selectively preserved, which is of great significance for the binarization of marble patterns on cow carcass eye muscles similar to texture images.
The application of backfat analyzer technology in grayscale image binarization is another image segmentation technique proposed by backfat analyzer. This method uses a hierarchical Gibbs random field model and a wavelet function to fit the mean surface in a hierarchical manner, achieving image binarization.
The backfat analyzer is a binary threshold selection method based on wavelet analysis. By representing the feature points of the image histogram from coarse to fine, a limited number of valley points are determined as candidate threshold points at the optimal scale. Then, an improved Otsu method that reflects the properties of the threshold points is used to identify a unique threshold point. This method is computationally simple, highly adaptable, and meets the real-time requirements of beef image processing and grading systems.
The backfat analyzer compared binary algorithms based on the maximum variance threshold method, the gray expected value method, and an improved local threshold method. Using the above methods, the R array, G array, and B array of the image were separately binary processed in RGB format, and then the result arrays were subjected to "OR" operations as binary results. This method can provide optimization algorithms for the segmentation processing of actual beef images, and the research on the segmentation technology of beef carcass eye muscle images using backfat analyzer has significant reference significance.
link: https://www.bxlimage.com/nw/685.html
tags: Backfat Cattle Carcass Eye Musclec Eye Musclec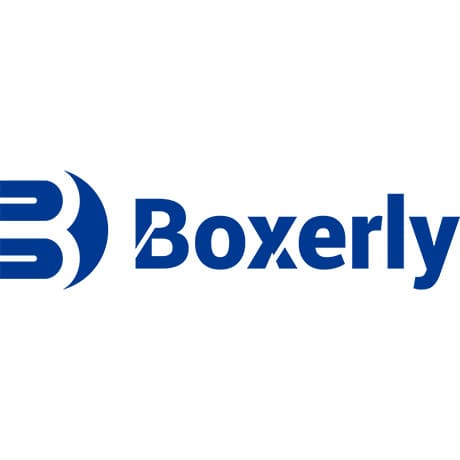
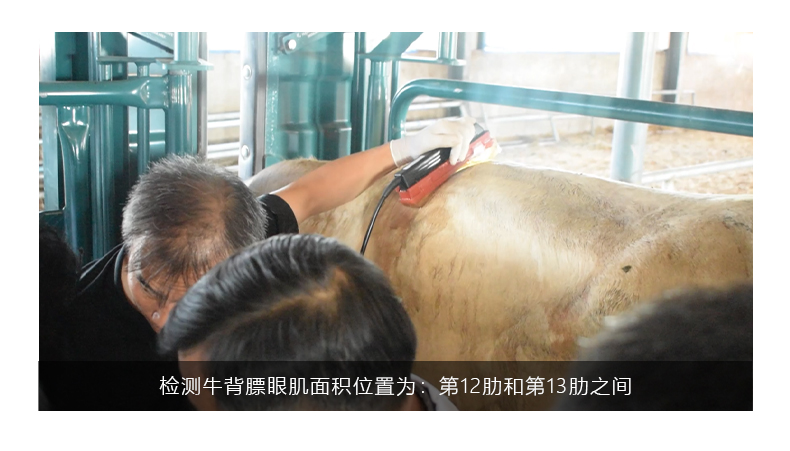

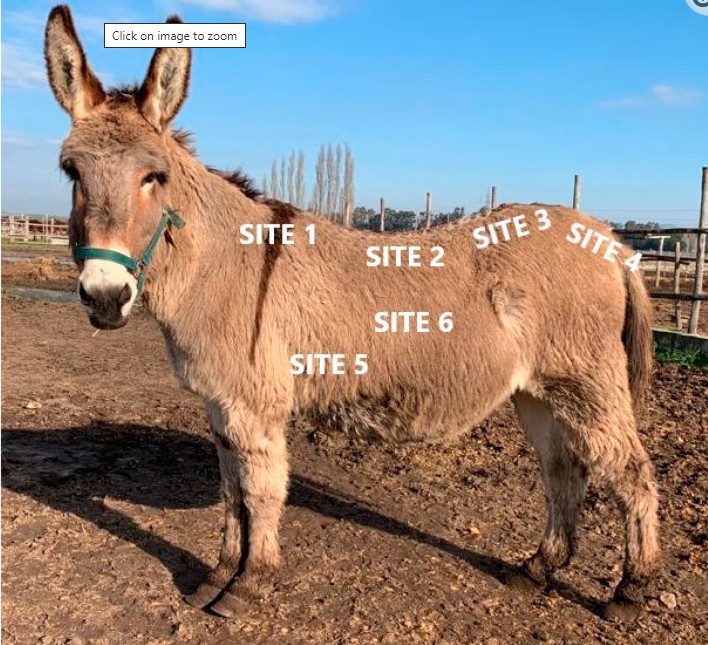